Nicholas C. Landolfi
Ph.D. Candidate, Computer Science Artificial Intelligence Laboratory (SAIL) Information Systems Laboratory (ISL) Stanford University For the immunologist (my father) see Nicholas F. Landolfi.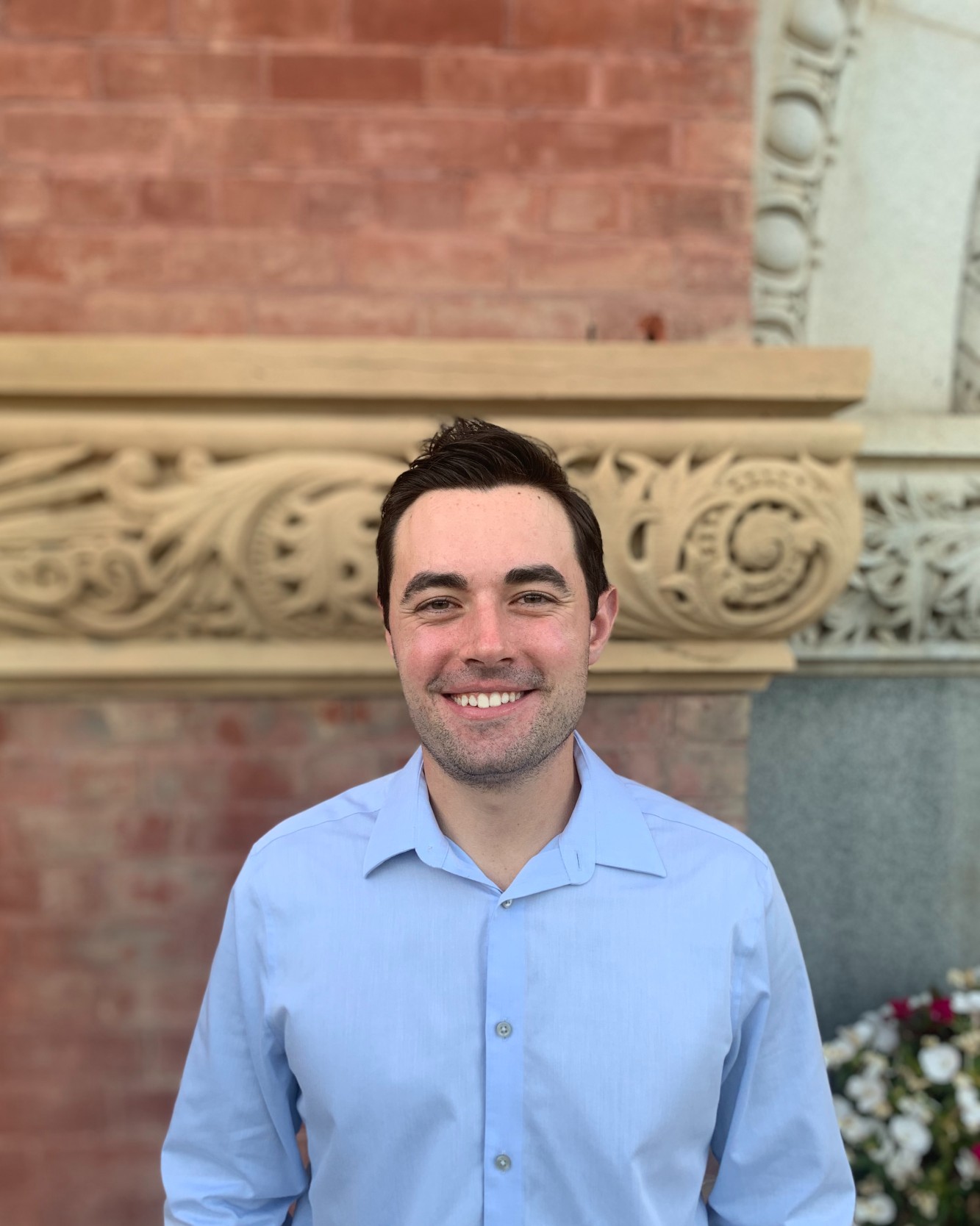
Bio
I am a Ph.D. candidate in the Computer Science department at Stanford.
I received a B.S. in Electrical Engineering & Computer Science with honors at the University of California, Berkeley in 2018.
My research focuses on algorithms for control, optimization and machine learning.
Teaching
Honors & Awards
Stanford Graduate Fellowship, 2021
National Defense Science and Engineering Graduate Fellowship, 2018
Regents' and Chancellors Research Fellowship, 2017
Arthur M. Hopkin Award, 2017
Regents' and Chancellor's Scholarship, 2014
Xerox Award for Innovation and Information Technology, 2014
Open Source
midGPT: simple hackable repo for FSDP pretraining 1B+ parameter base models
Papers
Optimal dorfman group testing for symmetric distributions
arXiv preprint, 2023 arXiv - bibtexUnsupervised language models for disease variant prediction
ML for Structural Biology and Learning Meaningful Representation of Life (Spotlight) Workshops, NeurIPS 2022. arXiv - PDF - talk - slides - posterProbabilistic Modeling Using Tree Linear Cascades
American Control Conference (ACC), June 2022 arXiv - talk - slides - longer slides - bibtex - github codeLearning Reward Functions from Diverse Sources of Human Feedback: Optimally Integrating Demonstrations and Preferences
The International Journal of Robotics Research (IJRR), 2021 arXiv - bibtexCloud Telemetry Modeling via Residual Gauss-Markov Random Fields
Conference on Innovation in Clouds, Internet and Networks (ICIN). March, 2021. PDF - bibtexA Model-based Approach for Sample-efficient Multi-task Reinforcement Learning
arXiv preprint, 2019 PDF - arXiv - bibtexAsking Easy Questions: A User-Friendly Approach to Active Reward Learning
Proceedings of the 3rd Conference on Robot Learning (CoRL), October 2019 PDF - arXiv - bibtexLearning Reward Functions by Integrating Human Demonstrations and Preferences
Proceedings of Robotics: Science and Systems (RSS), June 2019 PDF - arXiv - bibtexSocial Cohesion in Autonomous Driving
International Conference on Intelligent Robots and Systems (IROS), 2018 PDF - arXiv - bibtexPlanning for Cars that Coordinate with People: Leveraging Effects on Human Actions for Planning and Active Information Gathering over Human Internal State
Autonomous Robots (AURO), 2018. PDF - bibtexExploring Active Human Goal Inference in Shared Autonomy and Autonomous Driving
RSS Workshop: Mathematical Models, Algorithms, and Human-Robot Interaction, 2017. PDF - bibtexTalks
midGPT @ Innovation Endeavors (Dec. 2023)
Unsupervised Language Models for Disease Variant Prediction (Dec. 2022)
Efficient Disease Screening Using Group Testing and Symmetric Probability (Oct. 2022)
PCA 2 Ways (for teaching EE263) (Aug. 2022)
Tree Linear Cascades @ ACC (Jun. 2022)
Probabilistic Modeling Using Tree Linear Cascades (Apr. 2022)
Latent Variable Models for Genomic Data (Mar. 2022)
Basic mathematical genomics (Jan. 2022)
Dorfman-Rosenblatt Testing (Dec. 2021)
Tree Densities (Mar. 2021)
Cloud Telemetry Modeling (Mar. 2021)
Tree Distributions (Jan. 2021)
Directed Information (Oct. 2020)
Causal Models (Jul. 2020)